Regresión Logística para clasificación en R
datos = read.csv("Social_Network_Ads.csv", sep = ",", dec = ".", header = T)
print(head(datos))
User.ID Gender Age EstimatedSalary Purchased
1 15624510 Male 19 19000 0
2 15810944 Male 35 20000 0
3 15668575 Female 26 43000 0
4 15603246 Female 27 57000 0
5 15804002 Male 19 76000 0
6 15728773 Male 27 58000 0
str(datos)
'data.frame': 400 obs. of 5 variables:
$ User.ID : int 15624510 15810944 15668575 15603246 15804002 15728773 15598044 15694829 15600575 15727311 ...
$ Gender : chr "Male" "Male" "Female" "Female" ...
$ Age : int 19 35 26 27 19 27 27 32 25 35 ...
$ EstimatedSalary: int 19000 20000 43000 57000 76000 58000 84000 150000 33000 65000 ...
$ Purchased : int 0 0 0 0 0 0 0 1 0 0 ...
Variables:
df <- datos[,c("Gender", "Age", "EstimatedSalary", "Purchased")]
print(head(df))
Gender Age EstimatedSalary Purchased
1 Male 19 19000 0
2 Male 35 20000 0
3 Female 26 43000 0
4 Female 27 57000 0
5 Male 19 76000 0
6 Male 27 58000 0
print(dim(df))
[1] 400 4
Variable y:
print(table(df[,c("Purchased")]))
0 1
257 143
print(table(df[,c("Purchased")])/nrow(df))
0 1
0.6425 0.3575
Variable categórica:
print(unique(df$Gender))
[1] "Male" "Female"
df$Gender <- factor(df$Gender,
levels = c(unique(df$Gender)),
labels = c(1,0))
print(head(df))
Gender Age EstimatedSalary Purchased
1 1 19 19000 0
2 1 35 20000 0
3 0 26 43000 0
4 0 27 57000 0
5 1 19 76000 0
6 1 27 58000 0
Ajuste modelo múltiple:
logistic <- glm(Purchased ~ Gender + Age + EstimatedSalary, data = df, family = binomial)
logistic
Call: glm(formula = Purchased ~ Gender + Age + EstimatedSalary, family = binomial,
data = df)
Coefficients:
(Intercept) Gender0 Age EstimatedSalary
-1.245e+01 -3.338e-01 2.370e-01 3.644e-05
Degrees of Freedom: 399 Total (i.e. Null); 396 Residual
Null Deviance: 521.6
Residual Deviance: 275.8 AIC: 283.8
summary(logistic)
Call: glm(formula = Purchased ~ Gender + Age + EstimatedSalary, family = binomial, data = df) Deviance Residuals: Min 1Q Median 3Q Max -2.9109 -0.5218 -0.1406 0.3662 2.4254 Coefficients: Estimate Std. Error z value Pr(>|z|) (Intercept) -1.245e+01 1.309e+00 -9.510 < 2e-16 * Gender0 -3.338e-01 3.052e-01 -1.094 0.274 Age 2.370e-01 2.638e-02 8.984 < 2e-16 * EstimatedSalary 3.644e-05 5.473e-06 6.659 2.77e-11 * --- Signif. codes: 0 '*' 0.001 '**' 0.01 '*' 0.05 '.' 0.1 ' ' 1 (Dispersion parameter for binomial family taken to be 1) Null deviance: 521.57 on 399 degrees of freedom Residual deviance: 275.84 on 396 degrees of freedom AIC: 283.84 Number of Fisher Scoring iterations: 6
Curva ROC:
Punto de corte:
Definiremos el punto de corte con la probabilidad del 70%.
Cada observación tiene una probabilidad asociada que fue ajustada por el
modelo logit, logistic$fitted.values
. Probabilidades mayores o
iguales que 0,7 será clasificadas como \(1\), es decir, que sí
compraron, las demás observaciones serán clasificadas como \(0\), no
compraron.
y_pred <- ifelse(logistic$fitted.values >= 0.7, 1, 0)
library(ggplot2)
ggplot(data = df, aes(x = Age, y = EstimatedSalary,
color = as.factor(Purchased), shape = Gender))+
geom_point(size = 3)+
labs(color = "Purchased")
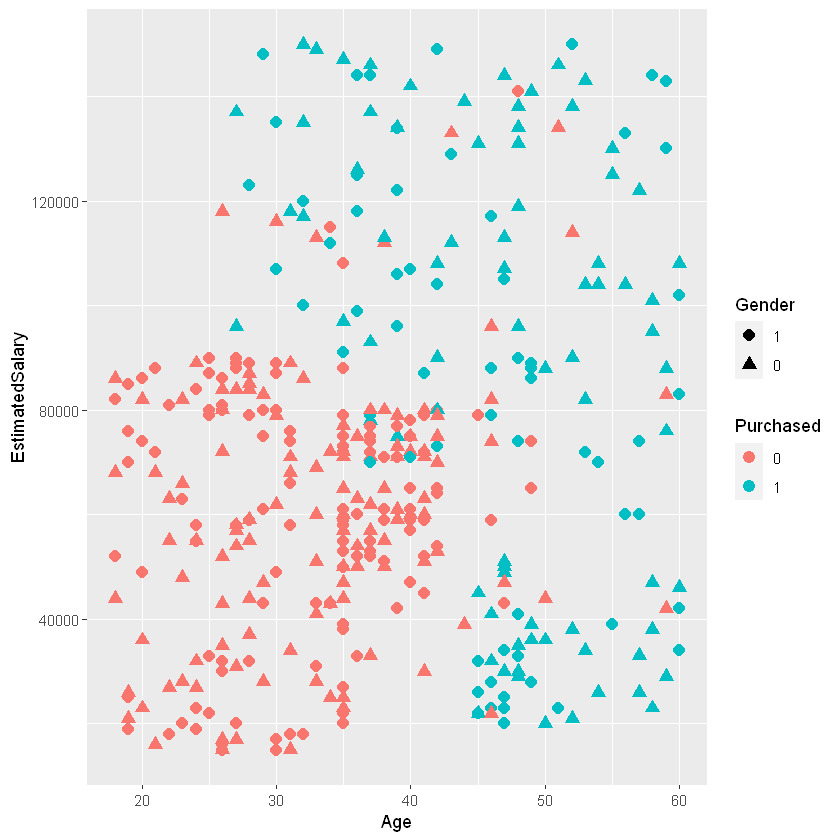
Instalar el siguiente paquete: install.packages('ROCR')
. Se usará la
función ROCR()
para graficar la curva ROC, pero primero se debe
crear un objeto de predicción, se usa la función prediction()
para
transformar los datos en un formato estándar que se pueda graficar con
la función ROCR()
. Luego, se crea un objeto con el rendimiento del
clasificador con la función performance()
, donde se agrega el
argumento measure = "tpr"
para indicar True positive rate y el
argumento x.measure = "fpr"
para False positive rate en el eje
\(x\) de la curva ROC.
library(ROCR)
Warning message:
"package 'ROCR' was built under R version 4.1.3"
prediction <- prediction(y_pred, df$Purchased)
perf <- performance(prediction, measure = "tpr", x.measure = "fpr")
plot(perf)
abline(a=0, b= 1)
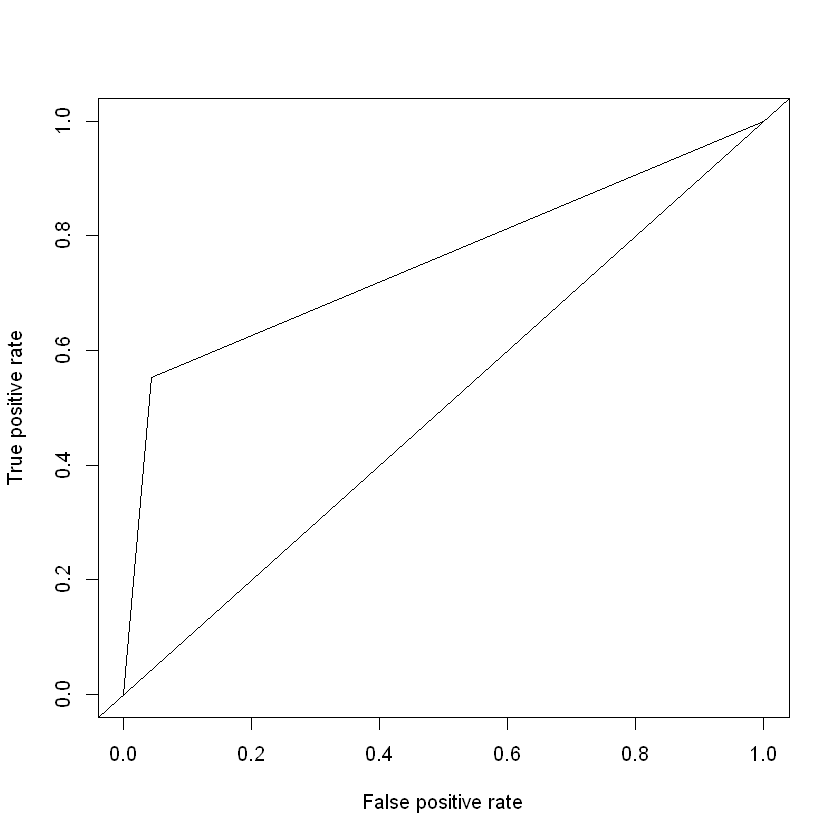
La anterior es la curva ROC para un punto de corto de 0,70.
AUC:
Podemos usar el paquete ROCR
para calcular el AUC. Para hacerlo,
primero debemos crear otro objeto de perf.auc
, esta vez
especificando measure = "auc"
.
perf.auc <- performance(prediction, measure = "auc")
Se usa @
porque el objeto creado tiene slots.
auc <- unlist(perf.auc@y.values)
print(auc)
[1] 0.754823
Matriz de confusión:
Antes de mostrar la matriz de confusión hagamos estos cálculos:
Cantidad de valores predichos como 1 (TP + FP):
print(sum(y_pred))
[1] 90
Cantidad de valores predichos como 0 (FN + TN):
print(length(y_pred) - sum(y_pred))
[1] 310
Cantidad de observaciones actuales que son 1:
print(sum(df$Purchased))
[1] 143
Cantidad de observaciones actuales que son 0:
print(length(df$Purchased) - sum(df$Purchased))
[1] 257
Predicciones correctas de la categoría 1 (Verdaderos Positivos - TP):
print(sum(ifelse(df$Purchased == 1 & y_pred == 1, 1, 0)))
[1] 79
Predicciones correctas para la categoría 0 (Verdaderos Negativos - TN):
print(sum(ifelse(df$Purchased == 0 & y_pred == 0, 1, 0)))
[1] 246
Predicciones correctas en total:
print(sum(ifelse(df$Purchased == y_pred, 1,0)))
[1] 325
Cantidad de predicciones incorrectas para la categoría 1 (Falso negativo - FN - Error tipo 2):
print(sum(ifelse(df$Purchased == 1 & y_pred == 0, 1, 0)))
[1] 64
Cantidad de predicciones incorrectas para la categoría 0 (Falso positivo - FP - Error tipo 1):
print(sum(ifelse(df$Purchased == 0 & y_pred == 1, 1, 0)))
[1] 11
La siguiente matriz de confusión tiene la forma:
Predicho como 0 |
Predicho como 1 |
|
---|---|---|
Actual 0 |
TN |
FP |
Actual 1 |
FN |
TP |
cm <- table(df$Purchased, y_pred)
print(cm)
y_pred
0 1
0 246 11
1 64 79
Métricas:
TP <- cm[4]
print(TP)
[1] 79
TN <- cm[1]
print(TN)
[1] 246
FN <- cm[2]
print(FN)
[1] 64
FP <- cm[3]
print(FP)
[1] 11
print(sum(cm))
[1] 400
Accuracy:
accuracy <- (TP+TN)/sum(cm)
print(accuracy)
[1] 0.8125
Error Rate:
error <- (FP+FN)/sum(cm)
print(error)
[1] 0.1875
Sensitivity:
sensitivity <- TP/(TP+FN)
print(sensitivity)
[1] 0.5524476
Specificity:
specificity <- TN/(TN+FP)
print(specificity)
[1] 0.9571984
Precision:
precision <- TP/(TP+FP)
print(precision)
[1] 0.8777778
Recall:
recall <- TP/(TP+FN)
print(recall)
[1] 0.5524476
F-measure:
F1 <- (2*precision*recall)/(recall+precision)
print(F1)
[1] 0.6781116
F1 <- (2*TP)/(2*TP+FP+FN)
print(F1)
[1] 0.6781116
¿Cómo cambian los resultados si el punto de corte es con una probabilidad de 0,50?